CT-IM FEATURES
Wrong-Way Detection with Deep Learning
Citilog’s Wrong-Way Detection with Deep Learning is an important tool within the CT-IM software suite, designed to accurately detect and alert traffic operators of wrong way driving incidents.
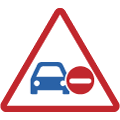
Superior wrong-way detection with deep learning
Wrong-way driving can result in severe accidents, particularly head-on collisions, and may cause secondary incidents as other drivers try to avoid the oncoming vehicle. Citilog’s system provides:
Real-time detection of wrong-way vehicles
The system instantly detects wrong-way vehicles and can proactively prevent accidents by automatically triggering warning systems to drivers.
Accurate classification of wrong-way incidents
Deep Learning helps classify the severity of incidents, allowing operators to prioritize responses and use resources efficiently.
Visual tracking for better operator insight
The system offers visual tracking, helping operators understand the vehicle’s path and quickly decide on appropriate actions to mitigate risks.
False alarm robustness
Citilog’s Deep Learning algorithms help reduce false alarms, which are a common issue in traditional detection systems, particularly in bidirectional traffic.
Wrong-way driving is a serious threat to road safety
Head-On Collisions
One of the most dangerous outcomes of wrong-way driving is the risk of severe head-on collisions, often resulting in serious injuries or fatalities.
Secondary Incidents
Drivers attempting to avoid wrong-way vehicles can cause additional accidents. Early detection minimizes these secondary risks.
Tunnel Safety
In confined areas like tunnels, quick detection is critical to prevent catastrophic incidents where escape routes are limited.
Not all wrong-way incidents are the same
Citilog’s system provides a clear understanding of each situation, enabling traffic operators to respond in a timely and appropriate manner. The system’s ability to differentiate between various wrong-way events improves safety and reduces the likelihood of secondary accidents.